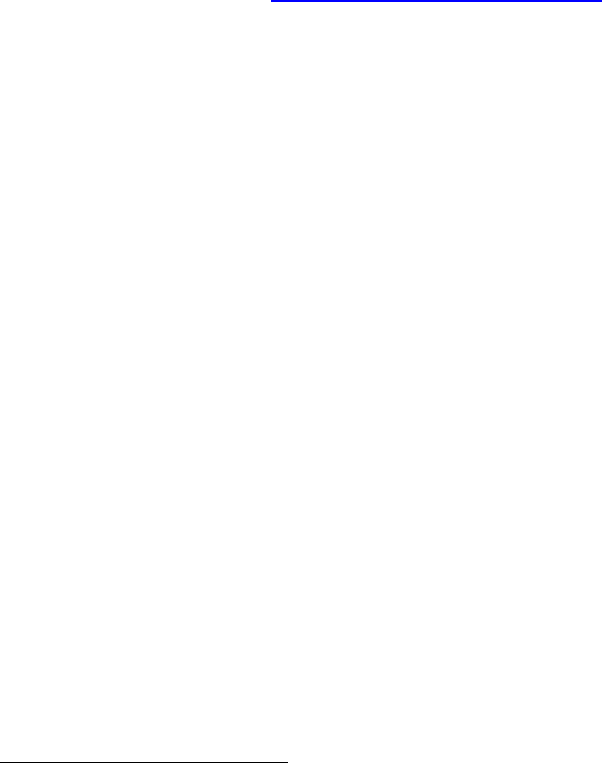
23
Faceapp!
71
are some examples of smartphone-based photo-editing applications that offer users
AI filters, backgrounds, and other effects to alter their photos.
In 2019, researchers significantly improved the image quality of generated faces,
72
demonstrating that computer generated faces can be nearly indistinguishable from photos of real
human faces on the website https://thispersondoesnotexist.com. Other tools and applications can
generate fake names, addresses, and assign fabricated data to other attributes, such as gender, age,
email, user ID, occupation, hobbies, and professional interests. Representative examples of these
applications include Faker
73
and Fake Person Generator.
74
In a more recent example, security
researchers have examined the proliferation of fake identities on professional networking sites by
generating fake names, resumes, and profile photos to target corporations and recruiters.
75
4.1.1.2. Impersonated Identity
An impersonated identity is an instance in which someone intentionally presents themselves as
someone else. Impersonated identity is a major factor in financial fraud, fraudulent loans, and
unauthorized use of credit. Credit card and payment fraud in the United States totaled an
estimated $9.62 billion in 2019 according to the Nilson Report.
76
This estimate does not include
fraudulent applications for government benefits and claims. Historically, fraud in unemployment
insurance has been largely attributed to issues of eligibility, misrepresentation of seeking work,
the terms of work separation, and unreported income. However, the Department of Labor
recently stated identity theft as a primary factor in recent fraud targeting unemployment benefits
during the pandemic.
77
The amount of improper unemployment payments related to the
pandemic is estimated to be in the billions of dollars.
78
In the cyber domain, the interception, theft, and unauthorized use of personally identifiable
information (PII) and identity credentials are enduring problems that enable cybercrime.
Phishing, business scams, and predatory romance scams frequently involve online impersonation
and use of false accounts. Targets typically receive an email that appears to originate from a
government agency or a reputable company, such as a financial institution, that encourages the
recipient to click on a link. This can redirect to a fake website made to look official that prompts
71
Bell, Karissa. 2019. “FaceApp Clones Are Also Going Viral, You Should Still Be Careful.” Mashable. July 18, 2019.
https://mashable.com/article/faceapp-alternatives-viral.
72
Karras, Tero, Samuli Laine, Miika Aittala, Janne Hellsten, Jaakko Lehtinen, and Timo Aila. 2020. “Analyzing and
Improving the Image Quality of StyleGAN.” IEEE Xplore. June 1, 2020. https://ieeexplore.ieee.org/document/9156570.
73
Faraglia, Daniele. 2020. “Joke2k/Faker.” GitHub. December 10, 2020. https://github.com/joke2k/faker.
74
“Fake Name Generator | Fake Person Generator.” n.d. Www.fakepersongenerator.com. Accessed August 23, 2022.
https://www.fakepersongenerator.com/fake-name-generator.
75
Krebs, Brian. 2022. “Glut of Fake LinkedIn Profiles Pits HR against the Bots – Krebs on Security.” Krebs on Security.
October 5, 2022. https://krebsonsecurity.com/2022/10/glut-of-fake-linkedin-profiles-pits-hr-against-the-bots/.
76
Robertson, David. 2020. Nilson Report. HSN Consultants, Inc.
77
Costa, Thomas, Mary Hannah Padilla, Seth J. Bagdoyan, Lawrance L. Evans, and Carol C. Harris. 2022. Unemployment
Insurance: Transformation Needed to Address Program Design, Infrastructure, and Integrty Risks. Washington DC: United States
78
Romm, Tony, and Yeganeh Torbati. 2022. "A magnet for rip-off artists: Fraud siphoned billions from pandemic
unemployment benefits." The Washington Post. May 15. https://www.washingtonpost.com/us-policy/2022/05/15/unemployment-
pandemic-fraud-identity-theft/.